Identifying Beliefs from Choice Data
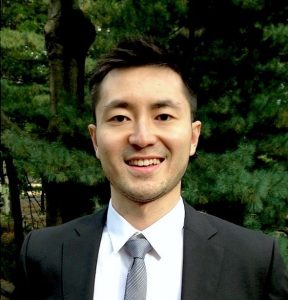
Jay Lu
How can we untangle beliefs from tastes in everyday decision-making? A hiring manager may reject a minority job applicant because he believes the candidate is unqualified or because of a personal bias against working with minorities. An admissions officer may reject a minority student because he believes the student is academically weak or because of prejudice toward minorities. Even a physician may recommend a certain drug to a patient because he believes it provides the best course of treatment or because of hidden financial incentives from the drug manufacturer.
In many of these decision-making environments, choices are made repeatedly across a large number of subjects and their frequencies aggregated in what is known as “stochastic choice data”. With the explosion of big data in recent years, such stochastic choice data has become increasingly available. In turn, this has spurred new research questions. What can we learn about people’s beliefs by analyzing such data? Does this particular form of data provide novel avenues of identification that are easier to use and employ? An active area of decision theory research has been trying to construct new tools and methodologies that make use of stochastic choice data for addressing these questions.
In ongoing research entitled “Bayesian Identification: A Theory for State-Dependent Utilities”, Professor Jay Lu studies how using information treatments with stochastic choice data can be used to differentiate between two forms of commonly observed discrimination: taste-based and statistical discrimination. For example, consider a hiring manager screening potential candidates from a minority group. In Becker-style models of taste-based discrimination, the manager hires less minority applicants due to some form of animus towards the minority group and is willing to pay a cost in order to avoid interacting with members of that group. On the other hand, in models of statistical discrimination, the manager hires less minority applicants due to stereotyping based on group membership that results from the manager’s imperfect information. While taste-based discrimination is prohibited, statistical discrimination is occasionally tolerated (e.g. older people being charged more for life insurance). When worker quality is not observed, the nature of discrimination is very hard to determine.
Can looking at changes in the manager’s information provide an answer? For instance, the recent rise in pre-employment job testing by employers provides an interesting comparative. After taking into account test scores in addition to interviews, the manager’s hiring should more closely reflect the true level of applicant quality. All else being equal, the true level of applicant quality would be lower under statistical than taste-based discrimination, so this would be reflected in the manager’s hiring. In short, since information affects beliefs but not tastes, the addition of more information would have different effects on hiring depending on the nature of discrimination.
There are many other situations where this methodology could be applied. For loan approvals, one could distinguish between taste-based or statistical discrimination by comparing approval rates before and after the introduction of automated credit scoring. For college admissions, the informational treatment could be the incorporation of in-person interviews. Moreover, the tools developed in this research could also be used for applications that do not involve discrimination. For medical advice, one may be worried that a physician is recommending a certain treatment due to financial incentives from the pharmaceutical company as opposed to concerns about patient well-being. In this case, the informational treatment could be the adoption of better diagnostic technology.
All these suggest that stochastic choice data could offer new methodologies for identifying beliefs and tastes in order to address important issues in regards to policy and regulation. Having a solid understanding of the connections between economic models and behavioral data not only helps to instruct us on how to conduct proper inferences but also provides insights on novel identification methodologies that may be easier or more efficient to implement.