Understanding Network Formation
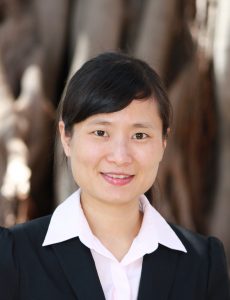
Shuyang Sheng
Social and economic networks permeate our lives. They play an important role in determining how diseases spread, which products we buy, how much education we attain, and even whether to smoke. Since social and economic networks are central to our economic well-being, economists have recently become increasingly interested in exploring how social and economic networks are formed. They raise empirical questions such as which network structures are likely to emerge, why some people have many friends while others are isolated, and whether people with similar socioeconomic status are more likely to become friends.
Answering such questions is challenging. Social and economic networks are not formed randomly. We choose our friends on the basis of the utility from friendships. When we make such decisions, we also take into account the other friends we already have or other friends our potential friends already have. For example, we are more likely to add someone as a friend on Facebook if we have friends in common. Such an effect of indirect friends creates strategic interactions among individuals, posing a big challenge to the econometric analyses of network data. Moreover, social and economic networks typically have hundreds or thousands of individuals. Since the number of network structures increases exponentially in the number of individuals, traditional econometric methods often become computationally intractable when networks are large.
In her ongoing research entitled “Estimation of Large Network Formation Games” joint with Geert Ridder (USC), Professor Shuyang Sheng develops new econometric methods to estimate network formation models with strategic interactions that overcome these challenges. Professor Sheng characterizes network formation as a simultaneous-move game with incomplete information which allows for utility externalities from indirect friends such as friends of friends and friends in common. Because the expected utility is nonlinear in the link decisions of an individual, each individual faces a complicated discrete choice problem with a large number of overlapping alternatives, which is difficult to solve. The insight of Professor Sheng’s work is to use the Legendre transform to express the expected utility as a linear function of the link decisions of an individual, so the optimal link decisions can be derived in closed form. Using this closed-form expression, Professor Sheng proposes a two-step procedure to estimate the parameters, which has good asymptotic properties and is easy to compute.
The methods proposed by Professor Sheng can be used to answer empirical questions in social networks such as how demographic and socioeconomic composition of students in a school affects their social networks, and whether friends in common play a significant role so the networks represent a feature of clustering. Besides social networks, the methods can also be used to investigate the formation of economic networks. For examples, citations are widely used to measure the economic value of a patent. Viewing patent citations as a network among inventors, one can use the methods to examine empirically why some patents receive many citations while other do not, whether inventors tend to cite patents whose inventors are not far from them, whether citation networks represent clustering and why, and so forth.