Should we regulate artificial intelligence?
By Joao Guerreiro
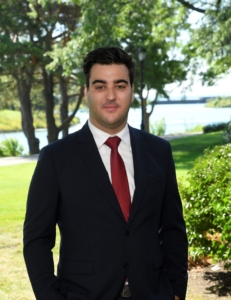
Joao Guerreiro
The short stories in Isaac Asimov’s “I, Robot” discussed some of the dilemmas associated with artificial intelligence (AI). New developments in AI technology have brought these concerns from the pages of science-fiction stories to the forefront of policy discussions. In May 2023, a global AI consortium declared AI risks a priority, similar to pandemics and nuclear war. The G7 started the Hiroshima AI Process for global AI regulation. Both the European Union and the U.S. are discussing regulatory frameworks. Ideas include mandatory testing, holding developers accountable, and classifying AI into risk tiers. The critical issue is the uncertainty surrounding AI’s societal costs and benefits.
How should AI be regulated in the presence of uncertainty regarding the AI’s potential adverse external effects? Professor Joao Guerreiro, with Sergio Rebelo (Kellogg School of Management) and Pedro Teles (Banco de Portugal and Catolica-Lisbon School of Business and Economics), tackles this question in a recent paper: “Regulating Artificial Intelligence.” The paper evaluates regulatory approaches using a normative analysis. The authors explore two settings. In the first scenario, uncertainty is only resolved after the release of the AI. In the second scenario, it is possible to beta-test the algorithm to assess external effects.
In the absence of beta testing, there’s a mismatch between the optimal AI novelty level for society and what naturally arises in an unregulated market. The paper argues that the social optimum, or the ideal balance of AI novelty and safety, is generally more conservative than what the market would select without regulation. With beta testing, developers can learn the external effects of the AI by testing before release. This approach helps resolve uncertainties regarding potential negative externalities. Still, the social optimum requires a higher degree of conservativism both in testing and the algorithm’s release.
The authors evaluate three regulatory frameworks. First, they show that subjecting algorithms to regulatory approval is insufficient to implement the social optimum– since developers still have the incentive to go for too-risky algorithms. Second, simply holding developers liable for the external effects of the algorithms is also insufficient to implement the social optimum in the presence of limited liability. Finally, they show that mandating beta testing to assess the externalities and holding developers liable for the adverse effects of the algorithm can achieve the social optimum, even in the presence of limited liability.
Overall, the paper’s findings highlight the complexity of AI regulation and the need for nuanced approaches that balance innovation and safety. By considering various scenarios and regulatory frameworks, it provides valuable insights for policymakers and stakeholders in the AI field.