Rethinking Randomized Controlled Trials
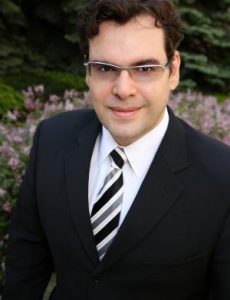
Rodrigo Pinto
Sir Ronald Fisher (1890-1962) is often regarded as the most influential statistician in 20th century. His 1935 book on “The Design of Experiments” sparked a revolution towards the use of the use of random assignments in assessing research inquiries. Fisher’s work is originally motivated by agricultural experiments. He explained how experiments that depart from the random assignments involve the judgment of the experimenter which leads to bias and inaccurate interpretation of data. In his own words: “If the design of the experiment is faulty, any method of interpretation that makes it out to be decisive must be faulty too.”
Economists have long benefited from Fisher’s ideas. Randomized Control Trials are often considered the gold standard for evaluating causal effects in social experiments. In it, individuals are randomly assigned to treatment groups and, if the RCT is perfectly implemented, then the average causal effect of the treatments on an outcome can be evaluated by comparing the outcome data across treatment groups. Unfortunately, perfectly implemented Randomized Control Trials are rare in social experiments, and most experiments struggle with some degree of noncompliance, whereby agents choose not to comply with its original treatment assignment. This is a particular problem with a variety of experiments on early childhood education such as the Abecedarian project, Head Start, the Educare program and the Perry Program.
Noncompliance induces selection bias that prevents the evaluation of the causal effects intended by the RCT. Faced with this caveat, experimental economists seek strategies that prevent noncompliance while econometricians have developed statistical methods to correct for noncompliance. These efforts share the same mindset: the original treatment assignments of the Randomized Control Trial is a desirable benchmark and deviations from it ought to be avoided.
Professor Rodrigo Pinto’s recent work reverses this mindset. His paper on “Randomization Biased-Controlled Experiments” is built upon a simple insight: while a departure from random assignments in an agricultural experiment is a failure of the experiment, a departure from random assignments in a social experiment is a realization of a rational choice and, thereby, a useful source of information.
Randomization Biased-Controlled Experiments is a novel framework that connects experimental design with a classical economic model to enhance causal inference. The method recognizes that a social experiment randomizes incentives instead of treatment choices. These incentives are the input of an economic choice model which employs revealed preference analysis to characterize the set of counterfactual choices that are economically justified. The economic model is then embedded into a casual model suitable to the study of treatment effects. The method innovates on standard RTC analysis by exploiting the information on the incentives induced by the experimental design. Moreover, depending on the design of incentives, noncompliance is not an econometric problem but rather an essential tool for the identification of causal effects.